WHAT DOES A DATA SCIENCE MANAGER DO?
Updated: Mai 19, 2025 - The Data Science Manager performs hands-on data exploration, processing, and analysis on massive datasets while utilizing machine learning, deep learning, and data mining techniques to solve complex business problems. This role involves pulling data, performing feature engineering, and developing state-of-the-art algorithms that uncover business opportunities from big data and deliver actionable insights to stakeholders. Additionally, the manager designs and implements advanced statistical and machine learning models, ensuring accurate results are consistently delivered in line with organizational guiding principles.
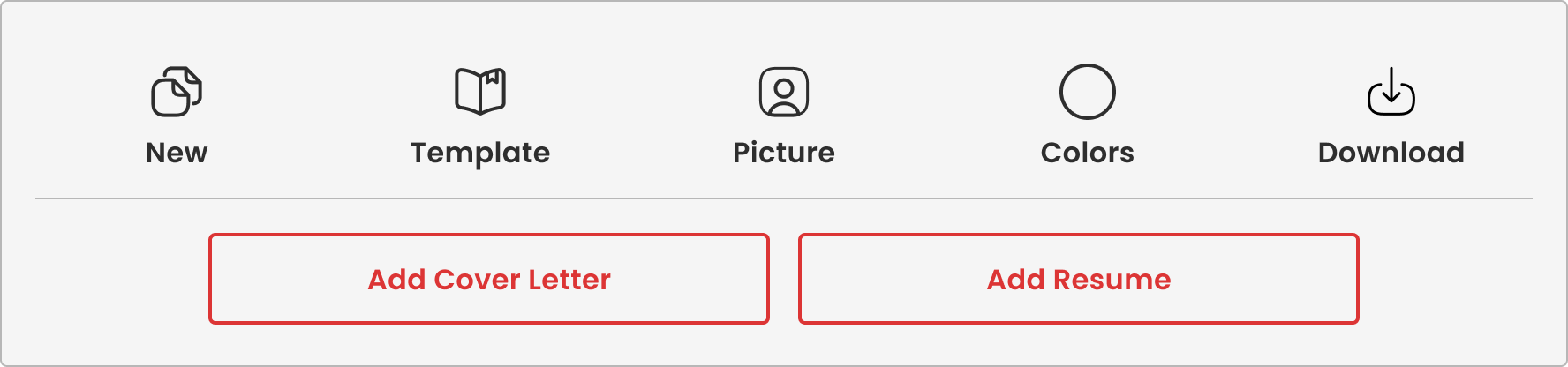
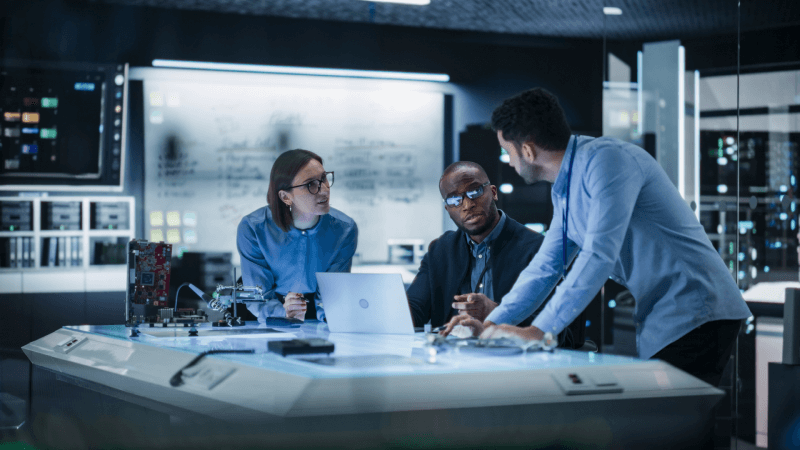
A Review of Professional Skills and Functions for Data Science Manager
1. Data Science Manager Roles
- Data Insight Translation: Work closely with divisions and departments to understand data insight needs and translate them into appropriate analytics instruments.
- Data Access Improvement: Improve data access processes enabled through the implemented technologies.
- Data Quality Monitoring: Develop processes and tools to monitor data quality.
- Business Performance Analysis: Develop processes and tools to monitor and analyze business performance.
- Data Architecture and Optimization: Architect, develop, and use a variety of datasets to find opportunities for product and process optimization.
- Analytic Instrument Development: Architect and develop a variety of analytic instruments (models, simulations, etc.) to test and validate the effectiveness of different courses of action.
- Data Leveraging: Work with stakeholders throughout the organization to identify opportunities for leveraging company data and non-structured domain knowledge to drive business solutions.
- Data Strategy Definition: Act as a key stakeholder in defining strategies for data capture, hygiene, and utilization.
- Analytics Management: Lead, define, and manage analytics releases, and build functional specifications for future analytics needs.
- Technical Design Representation: Represent the divisions and departments to ensure high-level technical design and project teams meet the defined schedules.
- Release Cycle Collaboration: Work with divisions and departments, applicable employees, and development teams during the design, development, test, training, and launch phases of the analytics release cycle.
- Quality Assurance: Ensure all customer and quality requirements are satisfied in the analytics product delivery.
2. Data Science Manager General Responsibilities
- Team Leadership: Lead a small team of data scientists and data engineers.
- Capacity Management: Manage demand and capacity.
- Opportunity Identification: Proactively seek demand opportunities.
- Process Design: Design and improve processes and methodologies to run the operation and to continuously enhance the delivery of the team.
- Training Development: Design training curricula for the team.
- Leadership Communication: Provide updates to leadership.
- Business Needs Analysis: Conduct/guide interviews with customers/requestors to interpret business needs.
- Stakeholder Engagement: Identify and connect with the right business SME applicable for each project.
- Workshop Facilitation: Drive workshops with the data science team and others (i.e., research) to reach the best approach for each project.
- Data Extraction and Transformation: Extract and transform data from data repositories.
- Statistical Analysis: Use descriptive and inferential statistics to explore and answer complex business questions.
- Predictive Modeling: Apply predictive modeling in either R or Python.
3. Data Science Manager Key Accountabilities
- Team Leadership: Lead a team of data scientists to leverage data and AI/ML to build data-driven products.
- Professional Development: Support the professional growth of the data science team through mentoring, coaching, and technical leadership.
- Vision and Roadmap Development: Build a vision and roadmap for the data science team and execute it.
- Cross-Functional Collaboration: Work with cross-functional partners in product, analytics, engineering, BI, marketing, finance, and consumer experience to identify trends, and new opportunities, and achieve business goals.
- Guidance and Support: Provide hands-on guidance to the team in building predictive models, developing machine learning solutions, designing experiments, and measuring impact.
- Technology Research: Research new technologies and methods across data science, NLP, computer vision, AI, and ML to improve the technical capabilities of the team and bring new process efficiencies.
- Project Management: Manage data science projects, track solution development, and deploy models in real-time and offline modes, as well as communicate results and performance to senior leadership.
- Stakeholder Engagement: Work closely with cross-functional stakeholders and the team to identify, lead, and deliver high-visibility machine learning solutions that improve members' experiences and contribute to business goals.
- Strategy Contribution: Contribute to data science strategy and roadmap and define technical excellence for data science at Chime.
- Mentorship: Mentor and develop team members, fostering a culture of innovation, collaboration, and continuous improvement.
- Talent Acquisition: Increase team impact by hiring high-caliber data scientists to solve the most impactful machine-learning problems.
- Requirements Management: Own data science requirements for the ML platform and work with ML engineers to deliver on shared objectives.
4. Data Science Manager Overview
- Predictive Modeling: Participating in the design and delivery of accurate and robust predictive models.
- Collaboration: Collaborating with Subject Matter Experts to design analytic solutions that enable better decision-making.
- Communication: Communicating findings, collecting feedback, and addressing questions and concerns, with the ability to translate technical concepts to a non-technical audience.
- Project Management: Managing large, long-term project timelines with cross-functional dependencies and transparently communicating progress on these timelines, obstacles, and solutions to any gaps.
- Statistical Modeling: Working with a team of data scientists to build out actionable statistical models (GLMs/ML/AI) within R/Python.
- Mentorship: Mentoring other data scientists on model development, best practices, technical coding, and analytics environment.
- Department Development: Assisting in the expansion and development of the analytics department, finding internal and external vendor partners to support long-term business objectives.
- Requirements Analysis: Determining requirements for the analytics platform.
- IT Collaboration: Collaborating with IT to deliver and maintain the environment.
- Machine Learning: Use machine learning to accelerate insights discovery.
- Data Visualization: Utilize data visualization to describe data findings in MS Excel, Power BI, or the tool of choice.
- Storytelling: Put together a story to articulate findings at a high level and present results to live audiences.
5. Data Science Manager Responsibilities
- Data Exploration: Perform hands-on data exploration, processing, and analysis on massive data sets.
- Machine Learning: Use machine learning, deep learning, and data mining techniques to solve business cases or problems.
- Feature Engineering: Pull data, perform feature engineering, back-test models, compare model performances, and communicate the results to stakeholders.
- Algorithm Development: Research and develop state-of-the-art algorithms that address complex business problems.
- Opportunity Identification: Identify and find business opportunities from big data via data analytic methods.
- Data Insights Advisory: Advise and recommend data insights to business users.
- Insight Discovery: Discover insights from massive amounts of structured and unstructured data to help shape or meet specific business needs and goals.
- Business Analysis: Analyze business problems, identify opportunities for improvement, and provide recommendations for optimal/efficient solutions.
- Result Delivery: Consistently deliver accurate results promptly.
- Model Development: Develop, validate, implement, and monitor statistical/machine learning models in a production environment.
- Guiding Principles Adherence: Consistently consider and follow ACA’s Guiding Principles.
- Data Modeling: Design and implement data modeling for solving specific and complex problems within business purposes by using advanced techniques of data science such as advanced statistics, mathematical models, and machine learning.