WHAT DOES A DATA QUALITY MANAGER DO?
Published: October 3, 2024 - The Data Quality Manager ensures the development and implementation of data quality standards by collaborating with stakeholders and translating business rules into actionable data governance strategies. Responsible for monitoring and improving data quality levels, the role includes managing compliance with data privacy regulations, identifying risks, and resolving critical data issues. This position also leads efforts in automating quality reports, implementing data cleansing processes, and providing guidance on data management best practices.
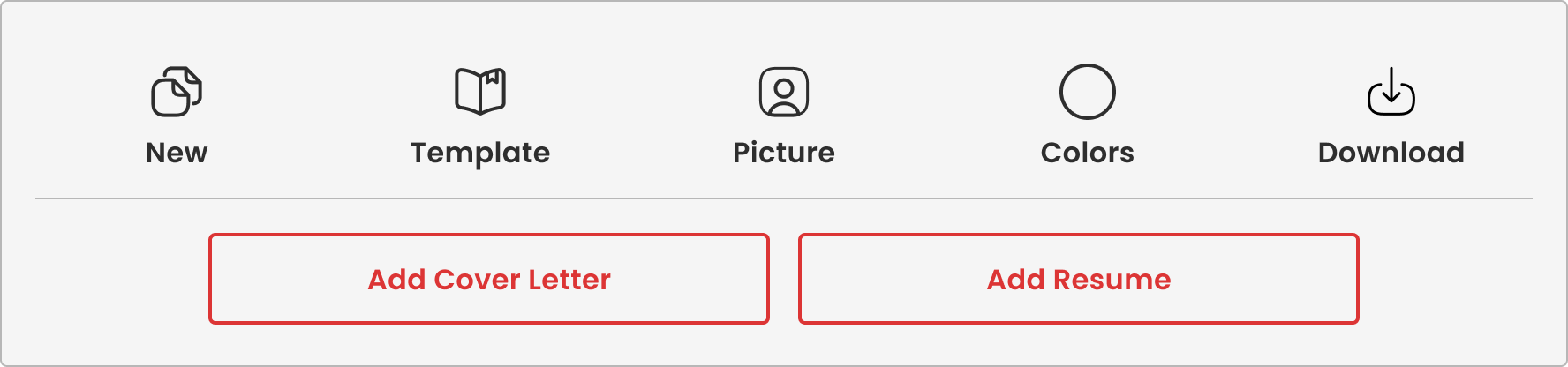
A Review of Professional Skills and Functions for Data Quality Manager
1. Data Quality Manager Duties
- Project Management: Take project manager role to go through SDLC activities for data analysis, cleansing, and data governance projects
- Stakeholder Collaboration: Liaise with business departments to define data quality business rules and data structure
- Data Cleansing Coordination: Conduct and coordinate users for data cleansing exercises
- Implementation Collaboration: Work with IT project teams and/or solution providers to implement data governance and quality rules
- Monitoring Compliance: Conduct ongoing monitoring of the conformance with the data quality management procedures and guidelines
- Technical Support: Provide production support and troubleshooting on data management systems
- Requirements Analysis: Analyze user and system requirements and set direction, guidelines, and procedures for data governance and data quality standards
- Presentations: Conduct presentations to business counterparts and users regarding data management issues
2. Data Quality Manager Details
- Data Quality Maintenance: Maintain existing Data Quality Framework by creating new data checks and refining existing data checks
- Project Participation: Participate in design and project planning sessions for data architecture on new projects and data flows, and define requirements for Data Quality
- Risk Identification: Identify what’s important for the business, what could break, and what needs rigorous checks
- Issue Resolution: Work with internal and external data partners on resolving data issues
- Process Improvement: Identify process improvements, data quality gaps, and implement data governance best practices
- Dashboard Management: Maintain existing Data Quality dashboard in PowerBI and advise the business on the overall state of data quality by regularly providing scorecards, metrics, and alerts
- Documentation: Document existing and potential data structures and data flow processes
- Vendor Collaboration: Work with vendors on new integrations and data exchanges, create data specifications, standards, and validation rules, and agree on data governance processes
- Data Coordination: Coordinate and test new data loads and data exchanges
- Error Troubleshooting: Troubleshoot data errors that fail processes or cause data quality issues
3. Data Quality Manager Responsibilities
- Data Quality Management: Manage the provincial data quality plan to enable better decision-making and health care planning
- Application Stewardship: Provide application stewardship and/or support to several of the ministries' enterprise applications
- Program Implementation: Implement recommendations to advance data quality programs within Alberta Health and Alberta Health Services
- Subject Matter Expertise: Provide subject matter expertise on patient activity reporting and grouping methodologies, CIHI products, diagnoses and intervention coding, and clinical vocabularies
- Information Management Promotion: Promote information management principles, practices, guidelines, and standards as they relate to data collection
- Educational Program Identification: Identify educational programs that will enhance the knowledge of provincial health information management professionals responsible for collecting and analyzing patient activity data
- Provincial Plan Leadership: Lead Alberta Health's implementation of the provincial data quality plan
- Improvement Identification: Identify and recommend data quality improvement opportunities to Alberta Health business areas
- Project Guidance: Guide project teams in incorporating data quality components in new project initiatives
- Issue Detection and Resolution: Manage the process for detecting data quality issues for corporate data assets and ensure corrective measures are implemented to prevent re-occurrence
- Team Guidance: Provide guidance and direction to staff on the data quality team, ensuring they are clear about their accountabilities and deliverables
- Standards Communication: Recommend and communicate approved national and provincial standard requirements for coding and classification of all clinical data
4. Data Quality Manager Job Summary
- Coding Standards Integration: Ensure that edits reflecting coding standards are built into data processing applications
- Standards Input: Provide input into proposed national coding and reporting standards requirements through the National Coding Advisory Committee
- Provincial Coding Alignment: Ensure all coding decisions are communicated provincially to align with CIHI's coding standards and direction
- SNOMED CT Leadership: Act as the provincial SNOMED CT education lead and provide input on initiatives related to potential provincial implementation of the clinical vocabulary for the Electronic Health Record
- Reporting Requirements Communication: Ensure data quality reporting requirements and responsibilities are communicated to Alberta Health Services facilities
- Provincial Network Leadership: Lead the provincial network responsible for quality data collection
- Strategic Guidance: Guide CIHI on the impact of strategic decisions concerning Canada's national health services databases through the National Clinical Administrative Databases Steering Committee
- Strategic Oversight: Provide strategic oversight and direction to Information Cross Functional Teams to ensure proper business processing of submitted records and timely availability of accurate patient activity data to the Ministry and regional health authorities
- Stakeholder Engagement: Build support for the acceptance of data quality strategy principles, approaches, and measures by engaging stakeholders in data quality initiatives
- Business Benefits Communication: Communicate business benefits for the adoption of corporate data standards
- Reporting Response: Respond to inpatient and ambulatory care reporting questions in a consistent and timely manner
5. Data Quality Manager Accountabilities
- Issue Resolution: Ensure issues are addressed with speed and thoroughness
- Requirements Collection: Collect requirements from data consumers and own validation rules that define data quality in a measurable way
- Team Management: Be responsible for the team (P&G IT Manager and an IT contractor) in their day-to-day operations, technical assessment, fixing, and follow-up on data quality issues
- Support Engagement: Engage and elevate issues to Data Engineering Support teams
- User Education: Carry out education sessions with users
- Cross-Team Collaboration: Lead and own interaction with different IT teams in the Programmatic space (Data Science, Data Engineering, Analysts, Platform teams), and IT Data Management horizontal owners (data operations, quality, security, and sourcing)
- Contract Collaboration: Collaborate with Purchasing to ensure Data Governance and Management best practices are implemented in contracts with data suppliers
- Innovation Leadership: Lead innovation, definition, and implementation of projects deploying standard data management solutions portfolio, including AI for data quality, Data Catalogue, and Data Lineage tools, and expanding data quality scope to other data types (e.g., publisher data)
- Data Quality Assurance: Collaborate with Data Engineering in charge of ETL processes to secure the highest quality of data and define the data transformation and harmonization logic
- Compliance Oversight: Ensure compliant archival, retention, privacy, and security policies are in place for each data type
- Risk Mitigation: Document and mitigate information security risks
6. Data Quality Manager Functions
- Data Quality Management: Manages the assessment, improvement, and governance of data quality at VNSNY
- Governance Rule Definition: Works with stakeholders to define governance rules used to develop and implement data quality standards
- Data Profiling: Profiles and analyzes data to develop insights in support of data governance and management activities
- Business Rule Translation: Works with IT developers to translate business rules into data quality rules and implement them
- Data Quality Monitoring: Monitors, tracks, and reports ongoing data quality levels
- Compliance Management: Ensures compliance with all applicable data privacy regulations and policies
- Target Setting: Establishes target goals for data quality improvement and identifies optimal approaches for resolving data quality issues to achieve targets
- Report Automation: Works with team members to produce and automate quality reports
- Collaboration: Collaborates and shares knowledge with internal teams to ensure a single source of truth for all data
- Issue and Risk Management: Identifies and manages critical data quality issues and risks
- Objective Execution: Prioritizes and executes a variety of objectives and clearly communicates progress and roadblocks
- Complex Concept Communication: Articulates complex concepts, thought leadership, and the business value of solutions in a clear and understandable way
- Data Cleansing: Develops and implements data cleansing processes to ensure foundational data and data models are complete, timely, and accurate
- Data Management Communication: Communicates the importance of managing data
- Staff Training and Evaluation: Ensures effective staff training, interviews candidates for employment, evaluates staff performance, and recommends hirings, promotions, salary actions, and terminations
7. Data Quality Manager Overview
- Standardization Leadership: Ensure a standardized and consistent approach relating to DM participation and implementation of Data Governance, Data Asset Management, Business Intelligence (BI), and Analytics
- Team Management: Manage, mentor, grow, and support a team of direct reports in the CDMO functional area
- Leadership Collaboration: Work within the leadership of CDMO to set personal and project goals, review and evaluate performance, and handle all aspects of human resource management within the assigned team
- Strategy Implementation: Manage day-to-day activities for the development of strategies, plans, tools, and approaches to ensure successful implementation of DM product coordination, governance, innovation projects, reports, dashboards, and scorecards
- Staff Coaching: Coach and mentor staff to think analytically and critically to lead data normalization, standardization, and automation efforts
- Effective Communication: Facilitate effective communication among all team members to ensure comprehensive understanding of needs, expectations, and requirements
- Data Quality Assessment: Lead the development of data quality assessments readiness for various activities and oversee data issue management
- Issue Resolution Oversight: Oversee issue gathering, definition activities, fact-finding, and resolution processes for the DM team
- Data Solution Development: Lead, design, and develop data solutions individually or through assigned team resources to meet organizational data business problems
- Cross-Departmental Communication: Ensure communication between project teams, analysts, developers, and all affected departments during data product build, implementation, and ongoing operationalization
8. Data Quality Manager Tasks
- Fiscal Responsibility: Exhibit fiscal responsibility by effectively managing department/project budgets
- Training Program Development: Develop and deliver staff training and education for trainers (train-the-trainer) and collaborate with vendors and internal resources to manage the delivery of data training programs/courses
- IT Collaboration: Collaborate with ITG in the definition and development of new or additional functionality to meet COG needs
- Success Criteria Definition: Participate in the definition of project success criteria and define metrics to be collected, analyzed, and reported
- Relationship Management: Establish and maintain effective relationships with COG, ITG, and other pertinent stakeholder professionals across the enterprise
- Issue Resolution: Proactively identify and resolve program-level issues
- Facility Support: Work on an as-needed basis with facilities that have hardships and opportunities for improvement with their data
- User Engagement: Engage data users to obtain feedback on usage activities to continuously improve DM output
- Data Evaluation: Use and support database applications and analytical tools, evaluate data quality, applications, and workflow functions
- Risk Escalation: Escalate to, and collaborate with, the CDMO Director on issues and risks to identify solutions
9. Data Quality Manager Roles
- External Collaboration: Responsible for leading work with external bodies, such as the Higher Education Statistics Agency (HESA), and the internal Data Management and Governance Team
- Data Quality Standards: Define and implement data quality standards and structures that address integrity, completeness, validity, uniqueness, accuracy, and consistency in data
- Data Governance Principles: Responsible for establishing data governance principles that address accountability, transparency, data protection, and compliance
- Standards Communication: Responsible for defining data standards, policies, and procedures, and clearly communicating them to Data Analytics colleagues, the wider organization, customers, and external bodies such as HESA
- Data Catalogue Management: Lead work on data catalog creation, data estate expansion, maintenance, and metadata management
- Governance Policy Development: Lead and contribute to the development of data governance policies and practices within Data Analytics and the wider organization
- Data Quality Audits: Lead work to establish processes to regularly review and audit data quality
- Compliance Oversight: Responsible for ensuring how we capture, process, share, recycle, and dispose of data complies with data protection and information security obligations
- Risk Management: Responsible for identifying, reviewing, and escalating data quality risks in line with the organization’s risk management approach
- Third-Party Data Processing: Assist colleagues in agreeing on how data is processed with third-party organizations, including discussions with Data Protection and Legal colleagues to ensure any processing of data is proportionate and legal
10. Analytics Data Quality Manager Additional Details
- Business Glossary Implementation: Drive the implementation and refinement of business glossary, data catalog, data lineage, and data quality capabilities to build trust and expand the understanding of analytics data assets
- Data Environment Expansion: Leverage and expand world-class data environment
- Information Management Support: Provide the foundation for information management and analytics supporting business intelligence, insights, and data science efforts
- Tool and System Enhancement: Maintain and enhance tools and systems related to data quality and knowledge management supporting enterprise analytics
- Data Integration: Direct efforts to combine disparate data, including complex data sets, structured and unstructured data, to proactively create value
- Process Optimization: Measure and optimize processes of data quality and metadata management to support production operations and project execution
- Technology Strategy Execution: Provide thought leadership and drive execution of the analytics technology data strategy
- Metadata Leadership: Lead metadata efforts to understand the business meaning of data and ensure data design aligns with development initiatives and standards
- Data Process Management: Manage and optimize processes for data intake, validation, mapping, semantic definition, modeling, visualization, and communication of deliverables
- Automated Quality Control: Lead data quality efforts to develop and implement automated quality controls and departmental standards to ensure quality standards, organizational expectations, and information security
- Quality Assurance Development: Develop quality assurance processes, alerts, and reporting to identify data integrity issues and work with owners to define processes to fix data quality issues at the source
- Testing Management: Manage efforts in the design and execution of test cases for all phases of testing, including system integration testing, user acceptance testing, performance testing, functional testing, operational testing, and deployment verification testing
- Automation Standards Definition: Define processes and standards for quality assurance automation efforts
- Team Leadership: Provide leadership, coaching, and mentorship to metadata and quality assurance team members
- Cross-Functional Collaboration: Collaborate cross-functionally with teams including engineering, business intelligence, architecture, insights, and data science to drive success
Relevant Information